Introduction
Demand forecasting plays a pivotal role in supply chain management, as it helps businesses to anticipate future customer demand and make informed decisions regarding inventory levels, production schedules, and resource allocation. This tutorial offers a comprehensive walkthrough of various demand forecasting techniques, including qualitative and quantitative methods, and provides practical guidance on selecting the most appropriate method for different supply chain scenarios. By understanding and implementing these techniques, supply chain professionals can improve the accuracy of their demand forecasts, minimize stockouts, and reduce costs.
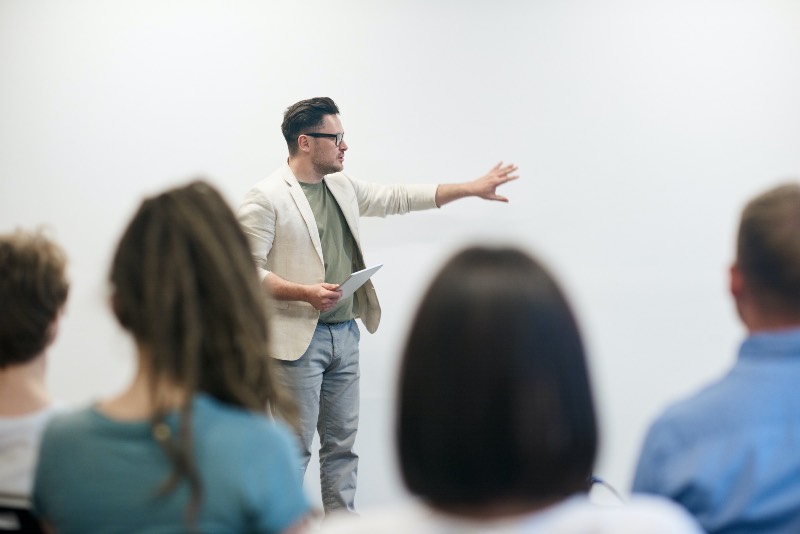
1. Qualitative Forecasting Techniques
Qualitative forecasting techniques are primarily based on expert judgment, market research, and the opinions of stakeholders, such as customers, suppliers, and sales representatives. These methods are particularly useful when historical data is scarce or when businesses need to factor in qualitative factors, such as market trends, competitive landscape, and economic conditions.
1.1. Delphi Method
Step 1: Assemble a panel of experts: Select a group of individuals with extensive knowledge and experience in the relevant domain.
Step 2: Distribute a questionnaire: Create a structured questionnaire containing open-ended questions related to the forecasting problem, and send it to the panel members.
Step 3: Compile responses: Collect and analyze the responses, summarizing the key insights and trends identified by the experts.
Step 4: Iterate: Based on the aggregated feedback, modify the questionnaire and send it back to the panel members for further input. Repeat this process until a consensus is reached.
Example:
A company that manufactures electric vehicles is planning to launch a new model and wants to forecast its demand. They gather a panel of experts with experience in the automotive industry, battery technology, and market trends. The panelists anonymously provide their individual demand estimates for the new model. The company then compiles these estimates and shares the aggregated results with the panel. The experts discuss the reasons for their predictions and re-submit their forecasts, taking into account the insights shared by others. This process continues until a consensus is reached, resulting in a more accurate and informed demand forecast for the new model. The additional insights from the iterative discussions among the experts help the company better understand potential challenges and opportunities associated with the launch of the new electric vehicle model.
1.2. Market Research
Step 1: Define research objectives: Identify the specific information that needs to be collected to support the demand forecasting process.
Step 2: Select research methods: Choose appropriate market research methods, such as surveys, interviews, focus groups, or observation, to collect the required data.
Step 3: Design data collection instruments: Develop structured or semi-structured questionnaires, interview guides, or observation forms to facilitate data collection.
Step 4: Collect and analyze data: Execute the chosen research methods, gather the data, and analyze the findings to identify trends, patterns, and insights related to future demand.
Example:
A fashion retailer wants to forecast demand for its upcoming clothing line. The retailer conducts market research through customer surveys and focus groups to gather insights on fashion trends, customer preferences, and potential demand for the new product line.
2. Quantitative Forecasting Techniques
Quantitative forecasting techniques rely on historical data and mathematical models to project future demand patterns. These methods are particularly useful when a business has access to a large amount of historical data and when the demand patterns are relatively stable and predictable.
2.1. Time Series Forecasting
Step 1: Collect historical data: Gather historical sales or demand data for the product or service in question, ideally over an extended period.
Step 2: Identify the components of the time series: Analyze the data to determine the underlying components, such as trend, seasonality, and cyclical or random variations.
Step 3: Select an appropriate model: Choose a suitable time series forecasting model, such as moving average, exponential smoothing, or autoregressive integrated moving average (ARIMA), based on the characteristics of the data.
Step 4: Forecast future demand: Apply the selected model to the historical data and generate forecasts for future periods.
Example:
A retailer wants to forecast the demand for winter jackets for the upcoming year. The retailer has five years of historical sales data available. They begin by analyzing the data and identifying any trends, seasonal patterns, or other recurring patterns. Through this analysis, they may find a consistent increase in demand year over year, as well as a seasonal pattern in the data. The retailer then applies an appropriate exponential smoothing model to forecast future demand, taking into account the identified seasonality. By incorporating these factors into the forecasting model, the retailer can more accurately predict future demand, allowing them to optimize their inventory levels and avoid stockouts or excess inventory costs.
2.2. Causal Forecasting
Step 1: Identify causal factors: Determine the factors that influence the demand for the product or service in question, such as price, advertising, promotions, or economic conditions.
Step 2: Collect historical data: Gather historical data for both the dependent variable (demand) and the independent variables (causal factors).
Step 3: Develop a statistical model: Use regression analysis or other suitable techniques to build a model that quantifies the relationship between demand and the causal factors.
Step 4: Forecast future demand: Apply the developed model to the forecasted values of the independent variables to predict future demand.
Example:
A manufacturer wants to forecast demand for its products, considering the impact of advertising expenditure and economic conditions. The manufacturer collects historical data on demand, advertising spending, and economic indicators, and develops a multiple regression model that captures the relationship between these variables. The manufacturer then uses this model to forecast future demand, given the planned advertising budget and projected economic conditions. By understanding the relationship between demand and the causal factors, the manufacturer can make more informed decisions about their advertising strategy and production plans, ultimately leading to improved supply chain efficiency and better alignment with market conditions.
3. Selecting the Appropriate Forecasting Method
Selecting the right forecasting technique is crucial for generating accurate and reliable demand forecasts. Supply chain professionals should consider the following factors when choosing a forecasting method:
– Data availability: Quantitative methods require historical data, while qualitative methods can be used in the absence of historical data or when qualitative factors need to be considered.
– Forecasting horizon: Short-term forecasts may benefit from quantitative methods, while long-term forecasts may require the use of qualitative methods that capture the potential impact of changing market conditions.
– Stability of demand patterns: If demand patterns are stable and predictable, quantitative methods may be more suitable. Conversely, if demand patterns are subject to significant fluctuations, qualitative methods may be more appropriate.
– Complexity of the forecasting problem: Simple forecasting problems may be addressed using basic quantitative methods, while more complex problems may require advanced quantitative techniques or the use of qualitative methods.
Conclusion
Demand forecasting is a critical component of effective supply chain management. By understanding and implementing various qualitative and quantitative forecasting techniques, supply chain professionals can improve the accuracy of their forecasts, leading to better decision-making and improved operational efficiency. This tutorial provided a comprehensive overview of demand forecasting techniques and offered practical guidance on selecting the most appropriate method for different supply chain scenarios. Check also our article on demand planning in supply chain management (http://supplychaintoolbox.com/supply-chain-planning/demand-planning-in-supply-chain-management/).
For more info on the method you can find following affiliate links on Amazon.
Check out this recommended resource on Amazon (affiliate link) for more information on the topic.
Geef een reactie