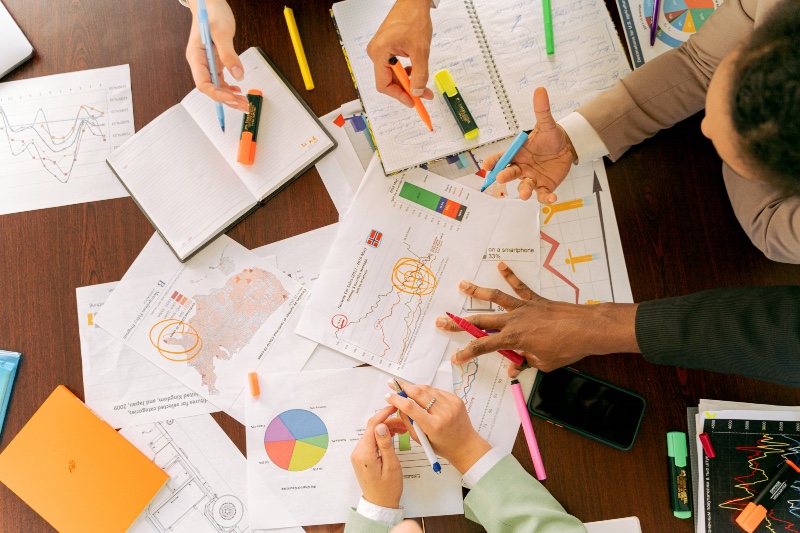
Introduction
In today’s competitive business environment, supply chain analytics plays a crucial role in driving data-driven decision making, improving operational efficiency, and gaining a competitive advantage. This article delves into the world of supply chain analytics, discussing essential techniques and tools, and providing a relevant case study. By implementing these best practices, supply chain professionals can transform their decision-making process and achieve better results.
The Importance of Supply Chain Analytics
Supply chain analytics is the process of collecting, analyzing, and interpreting data related to various aspects of a supply chain, such as procurement, production, transportation, and distribution. By utilizing data analysis techniques, businesses can identify patterns, trends, and correlations that can help them optimize their supply chain operations, reduce costs, improve service levels, and mitigate risks.
Key Techniques in Supply Chain Analytics
Descriptive Analytics: Descriptive analytics focuses on analyzing historical data to understand past performance and identify trends. This type of analysis can help businesses pinpoint areas of inefficiency, such as high inventory levels or frequent stockouts. This type of analytics is used to answer the question, “What happened?”
Example: A supply chain manager analyzes the previous year’s monthly sales data to identify seasonal patterns in demand. By understanding when demand typically increases or decreases, the manager can better plan for inventory needs and optimize warehouse space utilization.
Diagnostic Analytics: Diagnostic analytics involves examining data to determine the root causes of specific issues or problems. By understanding the underlying factors contributing to a problem, businesses can develop targeted solutions and strategies to address these issues. This type of analytics is used to answer the question, “Why did it happen?”
Example: A supply chain manager examines the reasons behind a sudden increase in lead times for a specific product. By analyzing factors such as supplier performance, transportation delays, and production bottlenecks, the manager can identify the root cause of the issue and implement corrective measures to prevent future delays.
Predictive Analytics: Predictive analytics uses statistical models and machine learning algorithms to forecast future trends and events based on historical data. This type of analysis can help businesses anticipate demand fluctuations, optimize inventory levels, and plan for potential disruptions. This type of analytics is used to answer the question, “What is likely to happen?”
Example: A supply chain manager uses historical sales data and advanced forecasting techniques to predict future demand for a product. By anticipating changes in demand, the manager can adjust inventory levels, production schedules, and transportation plans accordingly, reducing the risk of stockouts or excess inventory.
Prescriptive Analytics: Prescriptive analytics goes beyond forecasting to provide recommendations and actionable insights for decision making. By considering multiple variables and scenarios, prescriptive analytics can help businesses determine the best course of action to achieve their goals. This type of analytics is used to answer the question, “What should we do?”
Example: A supply chain manager uses a prescriptive analytics tool to optimize the company’s transportation network. The tool analyzes factors such as shipping costs, transit times, and delivery constraints, and recommends the most cost-effective and efficient transportation routes and modes for moving products from suppliers to customers.
By leveraging these different types of analytics, supply chain professionals can gain a deeper understanding of their operations, make data-driven decisions, and optimize their supply chain processes.
Case Study: Procter & Gamble
Procter & Gamble (P&G), a leading global consumer goods company, has successfully leveraged supply chain analytics to optimize its operations and drive significant cost savings. P&G implemented a robust data analytics platform that allowed the company to analyze data from multiple sources, including sales, operations, and external market data.
By applying advanced analytics techniques, P&G identified inefficiencies in its supply chain, such as excess inventory and redundant transportation routes. Using these insights, the company reduced its inventory levels by 25%, resulting in cost savings of over $1 billion. Additionally, P&G optimized its transportation network, reducing transportation costs by 15% and improving on-time delivery performance.
P&G’s success demonstrates the power of supply chain analytics in driving data-driven decision making and achieving tangible results.
Tools for Supply Chain Analytics
There are various tools available to help businesses implement supply chain analytics. Some popular options include:
Microsoft Excel: Excel is a widely used tool for data analysis, offering various functions and features for data manipulation, visualization, and basic statistical analysis.
Tableau: Tableau is a powerful data visualization software that allows users to create interactive dashboards and reports for easy data exploration and analysis.
R or Python: Both R and Python are popular programming languages for advanced data analysis, offering extensive libraries and packages for statistical modeling, machine learning, and data visualization.
IBM Cognos Analytics: IBM Cognos Analytics is a comprehensive business intelligence and analytics platform that provides advanced data analysis, visualization, and reporting capabilities.
SAP Analytics Cloud: SAP Analytics Cloud is a cloud-based analytics platform that combines planning, predictive analytics, and business intelligence features to help businesses make data-driven decisions and optimize their supply chain operations.
Best Practices for Data-Driven Decision Making
Centralize Data Collection: Collect and store data from various sources, such as ERP systems, CRM platforms, and external market data, in a centralized location to ensure data consistency and accessibility.
Invest in Data Quality: Ensure the accuracy and completeness of your data by implementing data validation processes, such as data cleansing and data enrichment, to improve the reliability of your analytics insights.
Foster a Data-Driven Culture: Encourage a data-driven mindset within your organization by promoting data literacy and providing training on data analysis techniques and tools.
Collaborate with Cross-Functional Teams: Engage stakeholders from different departments, such as sales, marketing, and operations, to share insights and collaborate on data-driven initiatives.
Continuously Monitor and Improve: Regularly review your analytics insights to inform strategic decisions, identify areas for improvement, and drive continuous optimization of your supply chain operations.
For more info on a critical aspect of supply chain management that relies heavily on data analysis and forecasting techniques, we would like to point to our article on Demand Planning (http://supplychaintoolbox.com/supply-chain-planning/demand-planning-in-supply-chain-management/)
In the ever-evolving world of supply chain analytics, several powerful tools can help businesses gain actionable insights from their data. For instance, IBM Cognos Analytics (https://www.ibm.com/products/cognos-analytics) offers a comprehensive suite of data visualization, reporting, and dashboarding capabilities that can simplify the analysis process. Similarly, SAP Analytics Cloud (https://www.sap.com/products/technology-platform/cloud-analytics.html) provides an integrated platform for business intelligence, planning, and predictive analytics, empowering organizations to make data-driven decisions with ease.
Geef een reactie