Introduction:
The emergence of Artificial Intelligence (AI) and Machine Learning (ML) has brought significant changes to various industries, and supply chain management is no exception. These technologies have the potential to revolutionize the way businesses optimize their supply chain operations, improving efficiency, reducing costs, and increasing agility. In this tutorial, we will explore the role of AI and ML in supply chain management, discuss their key applications, and provide examples to help you understand the practical implications of these technologies.
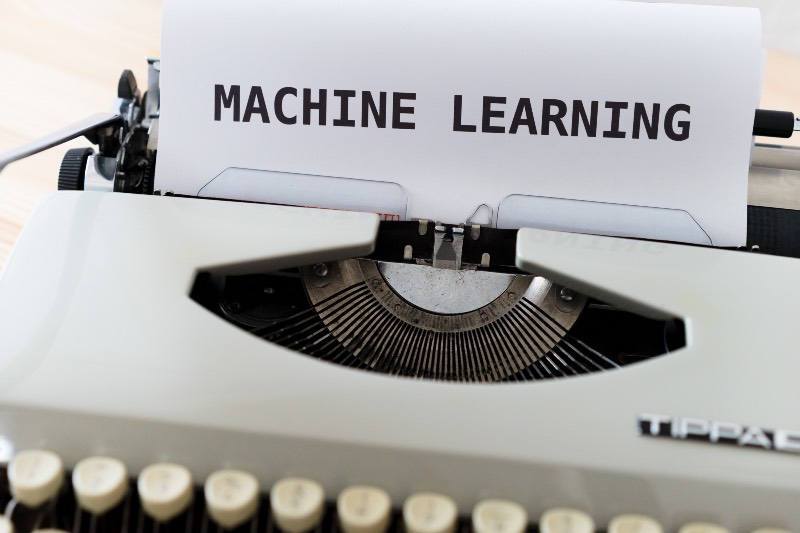
Table of Contents:
1. What is Artificial Intelligence and Machine Learning?
2. Key Applications of AI and ML in Supply Chain Management
- 2.1. Demand Forecasting
- 2.2. Inventory Optimization
- 2.3. Warehouse Management
- 2.4. Transportation and Logistics
- 2.5. Supplier Relationship Management
3. Examples of AI and ML Implementation in Supply Chain
- 3.1. Amazon’s Demand Forecasting and Inventory Optimization
- 3.2. IBM’s Watson Supply Chain Insights
- 3.3. Google’s DeepMind for Supply Chain Optimization
4. Challenges and Future Directions
5. Conclusion
6. Tips for Successfully Implementing AI and ML in Supply Chain Management
1. What is Artificial Intelligence and Machine Learning?
Artificial Intelligence (AI) refers to the development of computer systems that can perform tasks that usually require human intelligence, such as learning, reasoning, problem-solving, and decision-making. Machine Learning (ML), a subset of AI, involves the use of algorithms that allow machines to learn from data and improve their performance over time without explicit programming.
In the context of supply chain management, AI and ML can be used to analyze large volumes of data, identify patterns, and make predictions, which can help businesses make better decisions and improve their supply chain operations.
2. Key Applications of AI and ML in Supply Chain Management
2.1. Demand Forecasting
One of the critical applications of AI and ML in supply chain management is demand forecasting. By analyzing historical sales data, product attributes, and other external factors such as market trends and seasonality, AI and ML algorithms can generate accurate demand forecasts. This helps companies anticipate customer needs, optimize inventory levels, and reduce stockouts or overstocks.
Example: A retailer could use AI-powered demand forecasting to identify the most popular products during the holiday season, ensuring they have enough stock to meet customer demand without tying up capital in excess inventory.
2.2. Inventory Optimization
AI and ML can help companies optimize their inventory levels by analyzing factors such as demand, lead times, and stockout risks. By identifying the optimal safety stock levels and reorder points, businesses can minimize stockouts, reduce holding costs, and improve overall inventory efficiency.
Example: A manufacturing company could use ML algorithms to analyze historical demand data and production lead times, calculating the optimal safety stock levels for each product to minimize stockouts and reduce inventory holding costs.
2.3. Warehouse Management
AI and ML can also be used to optimize warehouse operations by automating tasks such as picking, packing, and sorting. For example, AI-powered robots can learn the most efficient routes for picking items, reducing the time it takes to fulfill orders. Additionally, AI and ML can help improve warehouse layout and space utilization by analyzing data on product dimensions, weight, and demand patterns.
Example: A distribution center could deploy AI-powered robots to automate the picking and packing process, increasing throughput and reducing labor costs.
2.4. Transportation and Logistics
AI and ML can play a crucial role in optimizing transportation and logistics operations. By analyzing data on shipping routes, transit times, and costs, AI and ML algorithms can help companies identify the most efficient and cost-effective shipping options. Additionally, AI-powered route optimization can help carriers reduce fuel consumption and emissions, contributing to more sustainable supply chain practices.
Example: A logistics company could use AI and ML to analyze real-time traffic data, weather conditions, and road closures, dynamically optimizing routes to minimize transit times and fuel consumption.
2.5. Supplier Relationship Management
AI and ML can help businesses better manage their relationships with suppliers by analyzing data on supplier performance, risk factors, and market conditions. By identifying the best suppliers, predicting potential disruptions, and proactively addressing issues, companies can minimize risks and ensure a more resilient and responsive supply chain.
Example: A company could use AI-powered supplier risk assessment tools to analyze data on supplier financial health, delivery performance, and other risk factors, helping them identify potential supply chain disruptions and take appropriate action.
3. Examples of AI and ML Implementation in Supply Chain
3.1. Amazon’s Demand Forecasting and Inventory Optimization
Amazon has long been a pioneer in the use of AI and ML in supply chain management. One of the key ways Amazon leverages these technologies is by optimizing demand forecasting and inventory management. By analyzing vast amounts of data, including customer browsing history, purchasing patterns, and external factors like weather and events, Amazon can generate highly accurate demand forecasts. This helps the company optimize inventory levels, reducing stockouts and overstocks, and minimizing costs.
3.2. IBM’s Watson Supply Chain Insights
IBM’s Watson Supply Chain Insights is an AI-powered platform that provides real-time visibility into supply chain performance, helping businesses identify and mitigate risks. The platform uses AI and ML to analyze data from various sources, including structured and unstructured data, to provide insights into supplier performance, potential disruptions, and areas for improvement. This helps companies make more informed decisions and proactively manage their supply chains.
3.3. Google’s DeepMind for Supply Chain Optimization
Google’s DeepMind, an AI research lab, has developed AI and ML algorithms that can be applied to various aspects of supply chain management, including demand forecasting, inventory optimization, and transportation planning. By leveraging deep learning techniques, these algorithms can learn from vast amounts of data and generate highly accurate predictions and insights, helping businesses optimize their supply chain operations.
4. Challenges and Future Directions
While AI and ML have the potential to transform supply chain management, there are also challenges and barriers to adoption. These include data quality and availability, the complexity of supply chain processes, and the need for skilled professionals who understand both the technology and the supply chain domain. Additionally, concerns around data privacy and security may also hinder the widespread adoption of AI and ML in supply chain management.
Despite these challenges, the future of AI and ML in supply chain management looks promising. As technology continues to advance and more businesses recognize the benefits of AI and ML, we can expect to see further innovation and adoption in the coming years.
5. Conclusion
In summary, AI and ML have the potential to revolutionize supply chain management by providing valuable insights, improving efficiency, and reducing costs. By implementing these technologies in areas such as demand forecasting, inventory optimization, warehouse management, transportation and logistics, and supplier relationship management, businesses can optimize their supply chain operations and stay ahead of the competition.
Relevant links:
1. IBM’s Watson Supply Chain Insights
2. Warehouse Automation: The Future of Supply Chain Management
6. Tips for Successfully Implementing AI and ML in Supply Chain Management
To maximize the benefits of AI and ML in supply chain management, companies should consider the following best practices:
6.1. Invest in Data Quality and Management
The accuracy and effectiveness of AI and ML algorithms rely heavily on the quality and availability of data. Investing in robust data management processes, including data cleansing, integration, and storage, will ensure that AI and ML models have access to the information they need to generate accurate insights and predictions.
6.2. Develop Cross-Functional Teams
Successfully implementing AI and ML in supply chain management requires collaboration between supply chain professionals, data scientists, and IT experts. By forming cross-functional teams, companies can ensure that all stakeholders understand the goals and objectives of AI and ML projects and can work together to achieve them.
6.3. Focus on Change Management
Introducing AI and ML in supply chain management can have a significant impact on existing processes and roles. To ensure a smooth transition, companies should develop a change management strategy that includes clear communication, employee training, and ongoing support.
6.4. Start Small and Scale Up
Instead of trying to implement AI and ML across the entire supply chain at once, companies should start with smaller projects that target specific pain points or areas of opportunity. This approach allows businesses to demonstrate the value of AI and ML, gain experience, and refine their processes before scaling up to larger initiatives.
6.5. Continuously Monitor and Improve
AI and ML models are only as good as the data they are trained on and the algorithms they use. Companies should continuously monitor the performance of their AI and ML models, making adjustments and improvements as needed to ensure ongoing success.
By following these best practices, businesses can successfully integrate AI and ML into their supply chain management processes, unlocking new levels of efficiency, cost savings, and competitive advantage.
Three highly recommended books on the subject of artificial intelligence and machine learning in supply chain management are:
1. “Innovative Supply Chain Management via Digitalization and Artificial Intelligence” by Chiranji Lal Chowdhary (https://amzn.to/3Jxgb1E) This book provides a comprehensive overview of AI applications in supply chain management, addressing various challenges and discussing key trends in the field. It covers both theoretical and practical aspects of AI, making it suitable for both academics and practitioners.
2. “Machine Learning, Blockchain, and Cyber Security in Smart Environments: Application and Challenges” by Dr. Sarvesh Tanwar (https://amzn.to/3uW7p8d) This book focuses on the application of machine learning techniques for demand planning and forecasting in supply chain management. It offers practical guidance on how to design, build, and implement effective machine learning models to improve forecasting accuracy and optimize inventory management.
These books will provide valuable insights and practical guidance on how to leverage artificial intelligence and machine learning to transform supply chain management and unlock new opportunities for growth and efficiency.
Geef een reactie